TL;DR — The recent release of performance targets for low-cost sensors by the United States Environmental Protection Agency (USEPA) highlights the important role that calibration plays in the effective use of data from these devices. Correcting the measurements collected by low-cost sensors with a calibration model that takes local environmental conditions and other variables into account helps the data from these networks meet performance targets. In turn, the publication of a standardized approach to interpreting data from low-cost sensors by the USEPA supports the greater availability of reliable, real-time, and high-resolution air quality data around the world.
How calibration helps ensure that low-cost air quality sensor data meet performance targets
The USEPA’s performance targets and testing protocols provide the most standardized and comprehensive approach to benchmarking low-cost sensor (LCS) performance to date, and represent a significant step toward the development of a set of standards for LCS data accuracy. The development of such standards will further legitimize LCS for official uses and advance the availability of high spatiotemporal resolution air quality data worldwide.
Calibration is essential to the effective use of low-cost sensors in any air quality monitoring project — and is especially important when benchmarking low-cost sensor data performance against standardized targets like those released by the USEPA.
When thinking about LCS performance, it is important to consider that data accuracy can be assessed at multiple levels. When using low-cost sensors for non-regulatory supplemental and informational monitoring (NSIM), most users will be looking to deploy a network of LCS. If the manufacturer also includes services such as calibration, the final dataset produced by the solution may be very different from the raw data produced by the network of sensors themselves.

Even when LCS are factory calibrated, a regionally-tailored calibration model should be applied to further optimize for local conditions (like temperature, relative humidity, etc.) and pollutant profiles to obtain the most accurate measurements. Researchers have found that the main challenge for a large majority of LCS air quality monitoring projects is developing appropriate calibration models to ensure data quality. The results of our polling during our recent webinar on sensors in the urban environment also demonstrate that calibration and quality assurance/quality control (QA/QC) are seen as some of the most significant challenges to establishing an air quality monitoring network.

For particulate matter, calibration is especially important because particulate profiles (i.e., particle composition and size distribution) around the world vary based on pollutant sources and environmental conditions. Accuracy can be significantly improved to within the targets recommended by the USEPA through additional data processing with localized calibration models.
To demonstrate the importance of calibration to meeting the performance targets recommended by the USEPA, the following section includes the results from several example particulate matter calibrations of our Clarity Node-S sensors.
How calibrated data from low-cost sensors perform against EPA performance targets
Example Calibration Model Performance: Los Angeles, California
The dataset used for the plots below is from a Clarity device located in Los Angeles, CA with data collected from mid-February 2021 to mid-April 2021. The daily PM2.5 average concentrations observed by the reference monitors range from 2.95 to 26.38 µg/m³. This calibration did not precisely follow the EPA’s recommendations for performance testing given that three sensors were not collocated, but demonstrates how calibration can improve performance for an extended period of time (60 days).
While the raw sensor data met only two out of five of the USEPA’s recommended performance targets, the calibrated data was improved significantly for all specified metrics and met all five performance targets. This performance improvement is typical when comparing calibrated LCS data to uncalibrated data for the same period.

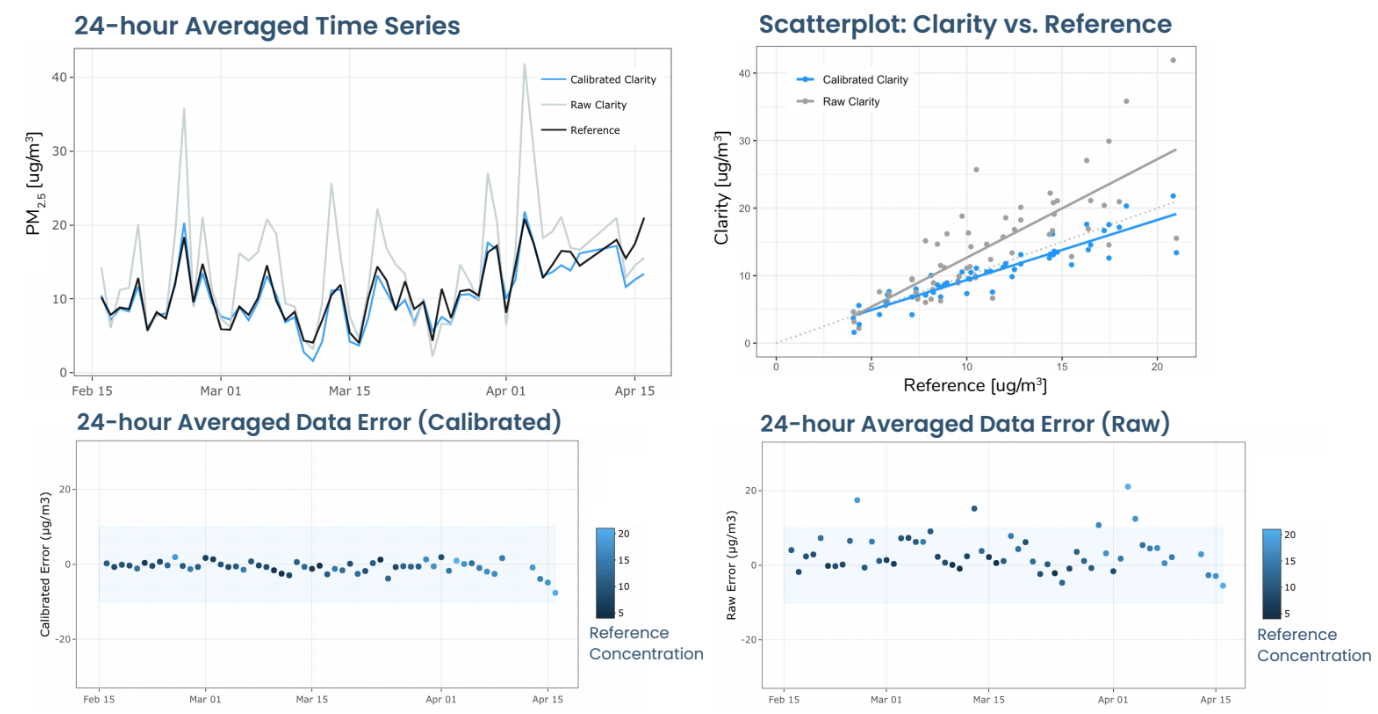
Example Calibration Model Performance: Phoenix, Arizona
The USEPA recommends performing field testing in two different climatic regions. To provide an additional example in a different climatic zone, we have included the results from the calibration of three of our Clarity Node-S sensors located in Phoenix, Arizona, here. As three sensors are included in this analysis, it is also more closely aligned with the testing protocols recommended by the USEPA.
The dataset used for the plots below is from three Clarity devices located in Phoenix, Arizona, with data collected from January 9, 2021, to February 11, 2021. The daily average concentrations observed by the reference monitors range from 2.86 to 35.02 µg/m³. Calibrations were applied on January 8th, 2021.
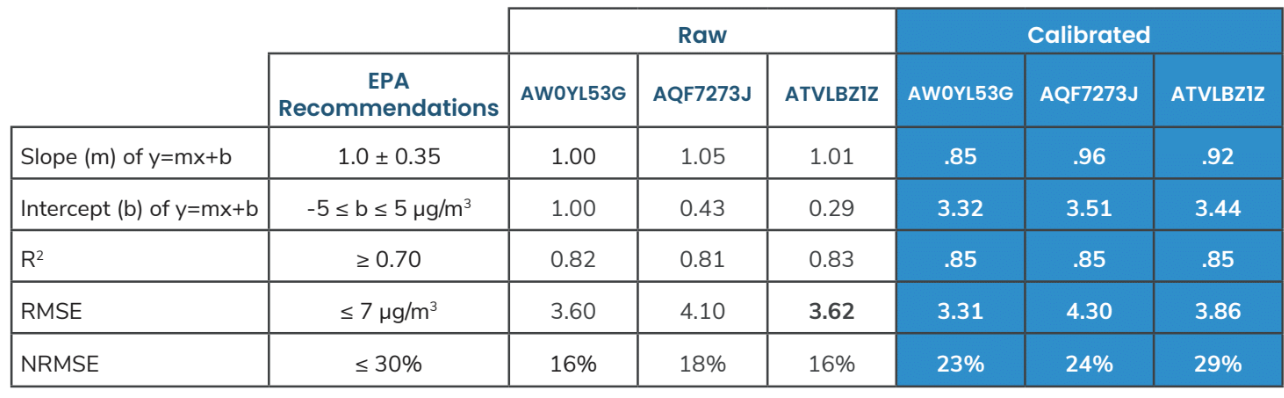

How to ensure data from your low-cost air sensors meets official performance targets
The availability of performance targets in no way guarantees that all commercially available LCS will meet those targets. Calibration is an important part of making effective use of LCS data and ensuring its accuracy, but not all air quality monitoring service providers provide calibration as part of their service offering.
It is of course possible to apply calibrations to your sensor network manually—but this is a process that requires a lot of overhead, not to mention technical expertise. Working with a reputable technology partner who includes calibration services helps to ensure that data from low-cost sensor networks meet USEPA and other performance targets.
Our take: Clarity’s Remote Calibration Model
At Clarity, we provide regionally-tailored calibration models for all of our partners through our Remote Calibration service. This process helps ensure the data from your networks meets the data quality standards recommended by the USEPA, the EU, MCERTS, and other regulatory bodies.
With Remote Calibration, our customers partner with Clarity’s Air Quality Experts to develop the best calibration model for their project, ensuring data is continuously adjusted to be as accurate as possible for local conditions. Clarity takes the lead on developing a regionally-tailored calibration model — meaning customers gain access to best-in-class sensor data with significantly less input or technical expertise required on their end. Once the calibrations have been applied, Clarity customers have access to both raw and calibrated data at all times in the Clarity Cloud.
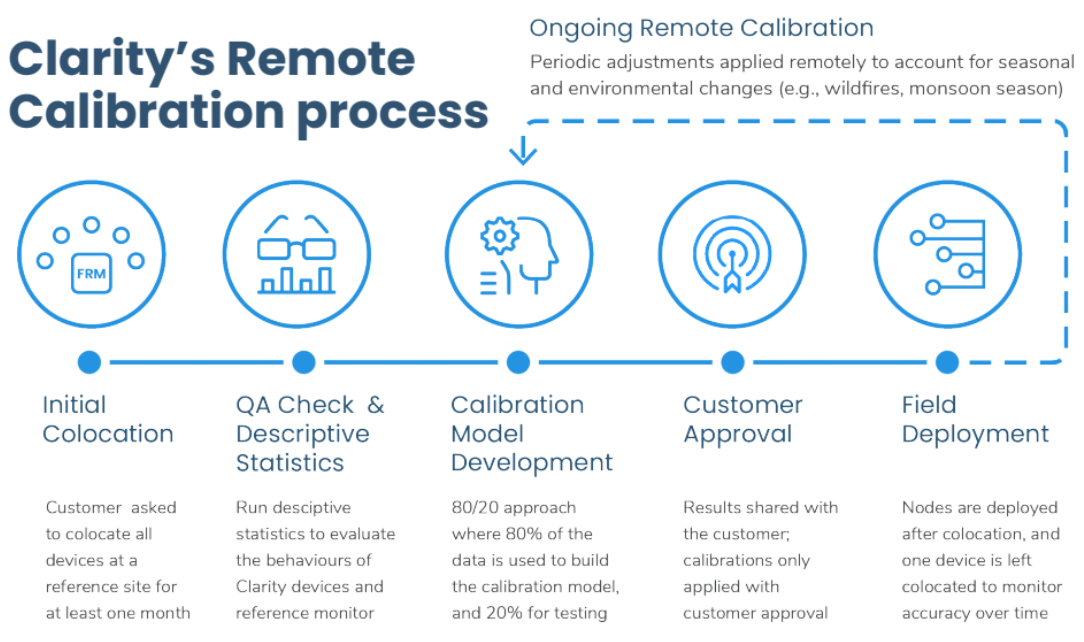
Want to learn more about how to properly calibrate low-cost air sensor data?
Low-cost air sensors are still a new technology that will continue to benefit from accuracy improvements as they see wider adoption by governments, communities, and businesses around the world. The USEPA’s publication of performance targets for low-cost sensors represents a significant step toward the development of standards that will enable the use of LCS technology for a wide range of applications.
The result will be better availability of high spatiotemporal resolution air quality data in near-real time for air quality managers, researchers, and the general public. The availability of this data will help governments and communities to better understand — and take action to reduce — air pollution around the world.
It is with this goal in mind that we provide Remote Calibration to our customers with sensor networks in 55+ countries around the world. To learn more about Clarity’s Remote Calibration process, check out our Guide to Accurate Particulate Matter Measurements with Air Sensors or contact us here to speak with an Air Quality Expert and learn more about our calibration process and results.