How to determine air quality sensor accuracy? What are the best metrics?
Accurate air pollution sensor data is vital in air quality monitoring. It helps us understand air pollution impacts and make informed decisions. Air quality sensors detect key pollutants such as PM2.5, ozone, and nitrogen dioxide, which affect human health. High levels of these pollutants contribute to respiratory and cardiovascular diseases, posing significant health risks.
Evaluating sensor performance involves understanding important metrics. These metrics ensure that the data collected is precise and reliable. Mean Absolute Error (MAE) is crucial in determining the difference between sensor readings and reference instruments. It highlights the need for accurate calibration practices. Combining MAE with the Pearson squared correlation coefficient (R²) offers a comprehensive assessment of sensor accuracy.
Precise data allows for effective pollution management and policy-making. By using reliable metrics, we can assess air quality accurately and address health risks. Clarity's expertise in air quality management supports these efforts, leveraging advanced sensor technology and data analytics. Our commitment to reducing global air pollution empowers communities to take action against environmental challenges. Understanding sensor accuracy is a step towards cleaner air and healthier communities.
Understanding key metrics for sensor accuracy
Evaluating air quality sensor accuracy helps ensure reliable data. Key metrics show how well sensors perform in capturing air pollutant levels.
- Mean Absolute Error (MAE): Measures the average magnitude of errors between sensor readings and reference instruments. Lower MAE values indicate higher accuracy, which means better air quality assessments. Detailed insights on assessing sensor accuracy using MAE can be found in our explanation on sensor accuracy using MAE.
- Root Mean Square Error (RMSE): Reflects the square root of the average of squared differences between predicted and observed values. RMSE is sensitive to larger errors, giving a fuller picture of sensor performance.
- R² correlation: Represents the squared correlation coefficient between sensor data and reference measurements. An R² value close to 1 indicates a strong relationship, highlighting the sensor's ability to track pollutant variations accurately. For more context on interpreting R² values, our assessment of sensor accuracy using R² offers further explanations.
Understanding these metrics helps determine the reliability of sensor data. MAE and RMSE focus on error magnitude, while R² assesses correlation strength. Each metric plays a key role in evaluating sensor performance, guiding improvements in air quality monitoring technology. These metrics help us improve our air quality solutions worldwide.
Challenges in ensuring sensor accuracy
Maintaining sensor accuracy in air quality monitoring presents several challenges. Environmental conditions like temperature and humidity can significantly impact sensor performance. Fluctuations in these conditions often lead to variability in sensor readings, making consistent accuracy difficult to achieve. For example, high humidity can cause particulate matter sensors to misread the concentration of pollutants, leading to inaccurate data.
- Calibration is crucial for ensuring sensor accuracy. Without proper calibration, sensors may drift over time, resulting in data that does not accurately reflect real-world conditions. Regular calibration against reference instruments is essential to maintain accuracy, yet it can be resource-intensive and requires technical expertise. For a detailed discussion on methodologies to assess sensor performance and the statistical measures involved, assessing air quality sensor accuracy offers further insights.
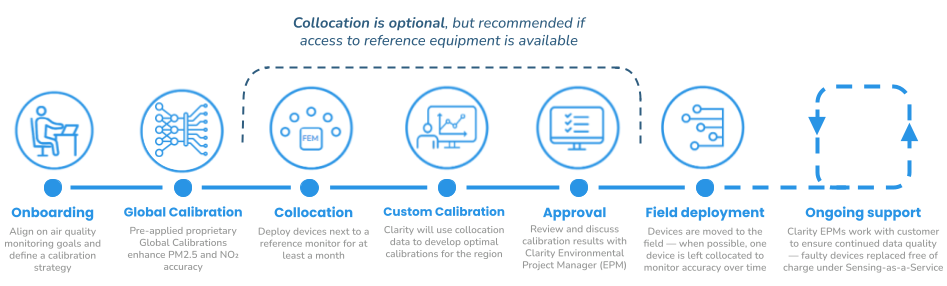
- External factors such as pollution sources and geographical location also affect sensor accuracy. Sensors placed near industrial areas or heavy traffic may experience interference from various pollutants, complicating the task of isolating specific pollutants for measurement. Additionally, sensors in different geographic locations may encounter unique environmental challenges, such as high altitudes or coastal climates, necessitating tailored calibration practices.
Clarity's approach to sensor accuracy includes leveraging advanced calibration techniques and robust sensor design to mitigate these challenges. By focusing on precision and reliability, Clarity's technology empowers users to confidently assess air quality, even in the face of environmental variability.
Methods for improving sensor accuracy
Enhancing air quality sensor accuracy involves specific techniques and best practices. Calibration that meets USEPA targets is fundamental. Regular calibration aligns sensors with reference instruments, ensuring precise readings. This process involves adjusting the sensor to match known standards, reducing deviations and improving reliability.
Collocation studies are crucial. Placing air quality sensors alongside regulatory-grade monitors reveals discrepancies and calibration needs. This allows for adjustments that enhance sensor performance in real-world conditions, ensuring data accuracy across diverse environments.
Onboard algorithms play a significant role. These algorithms process raw data to minimize errors, compensating for environmental factors like temperature and humidity. By filtering and correcting sensor output, algorithms enhance the precision of measurements, providing more reliable data for analysis.
Steps in a calibration process include:
- Identify reference standards: Select appropriate reference instruments for baseline comparisons.
- Initial calibration: Adjust sensors to align with reference standards, correcting initial deviations.
- Collocation testing: Deploy sensors alongside reference monitors to evaluate performance in specific settings.
- Iterative adjustments: Continuously refine sensor settings based on co-location results and observed discrepancies.
- Algorithm integration: Implement onboard algorithms to adjust for environmental variables, enhancing data accuracy.

Clarity's technology incorporates these methods, ensuring our sensors deliver high-accuracy data. By utilizing advanced calibration techniques, co-location studies, and onboard algorithms, we empower users with reliable tools for effective air quality management.
Evaluating the performance of low-cost sensors
Low-cost air quality sensors offer significant benefits in environmental monitoring. Their affordability and ease of deployment make them accessible options for areas lacking comprehensive infrastructure. However, their performance often diverges from traditional, higher-cost methods, necessitating careful evaluation to ensure accuracy.
The primary advantage of low-cost sensors lies in their ability to broaden air pollution monitoring coverage. They are instrumental in creating extensive networks that provide valuable data in real time. Yet, their limitations include potential compromises in precision and reliability. This makes rigorous assessment essential to verify their effectiveness against established standards.
Evaluating these sensors requires robust metrics to ascertain their accuracy and reliability. Employing standardized testing protocols is crucial for meaningful comparisons.
- Collocation testing: Positioning low-cost sensors alongside reference-grade instruments to identify discrepancies and calibration needs.
- Calibration practices: Regular adjustments to align sensor output with known standards, reducing deviations in data.
- Performance metrics: Utilizing measures such as Mean Absolute Error (MAE) and R² correlation to evaluate accuracy.
Understanding these techniques is vital for maximizing the utility of low-cost sensors. Integrating advanced calibration and evaluation methods ensures these sensors provide reliable data, supporting effective air quality management strategies.
Global standards and best practices for sensor evaluation
Global standards play a crucial role in air quality monitoring. They ensure consistency and reliability across diverse geographic locations. Standardized testing protocols are essential for evaluating sensor performance. They provide a framework for assessing accuracy, which is vital for building trust in air quality data.
International organizations have developed guidelines to facilitate these evaluations. For instance, learn more about collaborative efforts in global air quality monitoring that help shape these protocols. The United States Environmental Protection Agency (EPA) sets rigorous performance targets for air sensors, and its protocols guide the calibration and testing of air quality devices. These standards help maintain data integrity, ensuring accurate pollution assessments.
Adopting global best practices boosts confidence in air quality monitoring systems. Key initiatives include:
USEPA Performance Targets: Establish benchmarks for sensor accuracy, guiding consistent evaluations.
European Union (EU) Data Quality Objectives: Set standards for air quality assessment, influencing regional monitoring practices.
ISO Standards: Offer international benchmarks for environmental sensing and data management.
These initiatives highlight the importance of standardized practices in achieving reliable sensor data. Clarity adheres to these global standards, ensuring our solutions provide accurate and trustworthy air quality insights. For further context on how these standards integrate with advanced measurement technologies, see our discussion on air quality management 2.0 and the role of monitoring companies in accurate pollution measurement. By embracing these guidelines, we contribute to effective air pollution management worldwide.
Key takeaways on sensor accuracy metrics
Understanding air quality sensor accuracy is pivotal for effective environmental monitoring. Utilizing accurate metrics ensures reliable data collection, which is essential for informed decision-making and improving public health. Various metrics, such as Mean Absolute Error (MAE) and Root Mean Square Error (RMSE), offer insight into the precision of sensor readings. These metrics measure the magnitude of errors between sensor data and reference standards, providing a clear picture of sensor performance.
Pearson squared correlation coefficient (R²) is another vital metric, highlighting the correlation strength between sensor data and reference measurements. A high R² value signifies a strong predictive capability, essential for tracking pollutant variations accurately. These metrics form a robust framework for evaluating sensor accuracy, guiding enhancements in air quality monitoring technology.
For those seeking to implement advanced air quality solutions, explore the options available to build a tailored system. Discover how our expertise can support your monitoring needs by requesting a personalized quote.