Best practices for integrating air quality data with GIS
Integrating air quality data with GIS technology is essential for modern urban planning. This combination transforms raw data into actionable insights, offering a powerful tool for urban planners tasked with creating sustainable and resilient cities. Spatially aware, real-time data allows for precise decision-making, addressing challenges like lack of granularity and delayed responsiveness inherent in traditional monitoring systems.
GIS technology enhances the understanding of air pollution patterns by providing a visual representation of data across urban landscapes. This spatial context is crucial for identifying pollution hotspots, assessing the impact of urban development projects, and planning accordingly. Real-time integration ensures that data-driven decisions are made with the most accurate and current information, improving responsiveness to environmental changes and public health needs.
Benefits of integration air quality data with GIS data include:
- Enhanced Spatial Analysis: Utilize geographic contexts to pinpoint pollution sources and analyze trends over time.
- Informed Urban Planning: Enable planners to make data-backed decisions that support sustainable development.
- Improved Public Health: Identify and address critical areas to mitigate health risks associated with poor air quality.
- Efficient Resource Allocation: Direct resources to areas with the most significant need, optimizing interventions for maximum impact.
By leveraging the capabilities of GIS, urban planners can better manage air quality, ensuring healthier, more sustainable environments for communities.
Challenges in urban air quality monitoring
Urban air quality monitoring faces several challenges. Ground-based stations lack the spatial resolution needed to capture urban pollution complexity. These stations provide good data, but can't account for air pollution variability across different urban areas.
Pollution sources change constantly, affected by traffic, industrial activities, and weather. Ground-based stations can't deliver the detail needed for effective urban planning and policy.
Adding dynamic collection methods like low-cost sensors in urban environments and IoT devices improves data resolution. These technologies combined with GIS create a clearer picture of urban air quality. Our solutions include scalable air quality management tools that deliver the detailed insights cities need. With accurate, real-time data, urban planners can make better decisions for healthier, more sustainable cities.
The role of IoT in enhancing data collection
IoT sensors improve air quality data collection. These devices capture real-time, detailed data from across urban areas. They help us better understand air pollution, which is key for city planning and management.
Placing IoT sensors at multiple sites tracks air quality patterns. This network provides continuous data flow to monitor pollution levels and spot trends. Urban planners use this detailed information to make smart decisions for sustainable development and public health, as outlined in our discussion on the future of urban air quality management in large cities (air quality management 2.0).
Advantages of using IoT air quality sensors:
- Real-Time Insights: IoT sensors deliver current data, giving urban planners the latest information for decision-making.
- Granular data collection: These sensors gather detailed information from many locations, creating a complete air quality picture.
- Scalability: IoT networks expand easily as cities grow and change.
- Cost-effective solutions: IoT sensors cost less than traditional monitoring systems, making large-scale urban use practical. For deeper insights into deploying affordable sensor networks in urban areas, refer to our expert perspectives on low-cost air sensors in urban environments.

Our IoT technology gives urban planners tools to manage air quality proactively, creating healthier, more sustainable cities.
Integrating sensor networks with GIS platforms
Integrating air quality sensor networks with GIS platforms demands precise methodologies. Establishing data compatibility is crucial. Sensor data must align with GIS formats to ensure seamless integration. Leveraging standardized data protocols, such as GeoJSON or CSV, facilitates this alignment. This compatibility ensures that data from various sensors can be processed and visualized consistently within GIS systems.
Real-time data streaming is essential for dynamic urban environments. IoT sensors capture air quality data continuously, and integrating this data into GIS platforms requires robust streaming capabilities. This integration allows urban planners to access up-to-the-minute information, aiding swift decision-making. Implementing APIs enables automatic data transfer from sensors to GIS, maintaining the flow of real-time insights that are central to our air quality data management and analysis platform.
Visualization techniques enhance the understanding of air quality data within spatial contexts. Mapping tools within GIS platforms enable the creation of visual overlays that highlight pollution levels across different urban sectors, echoing the best practices for effectively communicating sensor data established by Clarity. These visualizations assist in identifying patterns and anomalies, supporting evidence-based planning and interventions.
Best practices for integrating sensor networks with GIS platforms include:
- Ensure Data Compatibility: Use standardized formats to align sensor data with GIS systems.
- Enable Real-Time Data Streaming: Implement APIs for continuous data flow into GIS platforms.
- Utilize Advanced Visualization: Leverage GIS mapping tools to create insightful visual representations of air quality data.
Clarity's expertise in integrating sensor networks with GIS platforms provides urban planners with powerful tools for managing air quality effectively. These methodologies support sustainable urban development and public health initiatives.
Data quality and calibration for accurate results
Accurate air quality monitoring relies heavily on data quality and precise calibration. Maintaining sensor accuracy—as detailed in our discussion on assessing air quality sensor accuracy assessing air quality sensor accuracy—is crucial for generating reliable insights that guide urban planning decisions. Calibration ensures that air quality sensors remain consistent and precise over time, adapting to the dynamic nature of urban environments.
Calibration techniques are vital in aligning air quality sensor data with data quality objectives and standards. This process involves comparing sensor readings with established benchmarks to ensure precision. Regular calibration helps in adjusting sensors to account for environmental changes and potential sensor drift, maintaining data integrity and reliability.
Key factors in properly calibrating air quality sensors include:
- Collocation: Place sensors alongside reference-grade monitors to identify and adjust discrepancies, a method supported by our detailed overview of calibration processes calibration process using collocation.
- Routine calibration checks: Schedule regular checks to maintain sensor accuracy and adapt to environmental shifts.
- Environmental compensation: Adjust sensors for temperature, humidity, and atmospheric pressure variations.
- Data validation: Cross-check sensor data with historical data and other monitoring systems for consistency.
Utilizing these calibration techniques ensures that air quality data is accurate and actionable. Clarity's expertise in sensor technology and data analysis supports urban planners in implementing these practices effectively. By prioritizing data quality and calibration, urban environments can achieve better air quality management and sustainable development outcomes.
Using predictive models for trend analysis
Predictive models are crucial in air quality management within urban planning, offering foresight and data-driven insights powered by robust air pollution data. Models like Convolutional Neural Networks (CNNs) and decision trees predict air quality trends, allowing urban planners to identify potential issues before they escalate. This capability aids in proactive urban management and supports sustainable development efforts.
CNNs excel in processing spatial data, making them ideal for analyzing complex air quality patterns across urban landscapes. They recognize patterns and anomalies in air quality data, helping planners anticipate pollution events and strategize interventions effectively. By leveraging CNNs, cities can maintain a continuous understanding of their air quality dynamics.
Decision trees offer simplicity and interpretability, essential for understanding decision-making processes in air quality management. These models categorize data based on specific criteria, facilitating clear predictions about air quality outcomes. Their straightforward nature enables urban planners to assess various scenarios and develop targeted strategies for pollution mitigation.
Some examples of predictive models that can be effective for air quality data include:
- Convolutional Neural Networks (CNNs): Analyze spatial data for pattern recognition in air quality trends.
- Decision Trees: Simplify decision-making with clear, interpretable predictions.
- Random Forests: Provide robust predictions by aggregating multiple decision trees.
- Support Vector Machines (SVMs): Classify air quality data for precise trend forecasting.
These predictive models empower urban planners with the tools to anticipate and manage air quality challenges effectively. More insights on integrating sensor networks and advanced monitoring techniques can be found in our discussion on collaborative efforts in global air quality monitoring, highlighting the benefits of uniting technological innovation with data analytics for healthier communities.
Real-world applications of integrated systems
Integrated IoT-GIS systems transform urban planning by providing dynamic, data-driven solutions. These systems are pivotal in optimizing traffic management, refining zoning adjustments, and enhancing disaster response strategies.
In traffic management, real-time air quality and traffic data guide urban planners in implementing adaptive traffic control measures. By integrating IoT sensors with GIS platforms, planners identify pollution hotspots caused by vehicle congestion. This data aids in devising strategies such as adjusting traffic signal timings or promoting alternative transportation routes to alleviate congestion and reduce emissions. For example, implementing adaptive controls can significantly lower vehicle emissions and urban air pollution through sensor monitoring strategies.
Zoning adjustments benefit from the detailed insights provided by integrated systems. These systems offer a comprehensive view of environmental conditions across different urban zones. Planners can assess the impact of proposed developments on air quality, ensuring zoning decisions support sustainable urban growth. By using GIS to visualize potential pollution impacts, urban planners make informed decisions that balance development with environmental health.
In disaster response, integrated IoT-GIS systems play a crucial role. They offer real-time monitoring of air quality conditions during events like wildfires or industrial accidents. This information helps emergency response teams allocate resources effectively and protect public health. By mapping affected areas, responders can prioritize evacuation routes and healthcare services, minimizing health risks for affected populations.
Some applications of integrated air quality and GIS systems include:
- Traffic Management: Implement adaptive traffic controls to reduce vehicle emissions and urban air pollution through sensor monitoring strategies.
- Zoning Adjustments: Ensure sustainable growth with data-driven zoning decisions.
- Disaster Response: Support emergency services with real-time air quality monitoring.
These applications of integrated systems empower urban planners to create resilient, sustainable urban environments. Urban planners can also explore detailed case studies on how integrated sensor networks support community resilience through effective air pollution measurement.
Addressing data integration challenges
Integrating air quality data with GIS platforms involves several challenges. Ensuring data format compatibility, maintaining sensor coverage, and managing large datasets demand precise methodologies.
Data format compatibility is crucial for seamless integration. Sensor data needs to align with GIS formats to ensure consistency and usability. Using standardized data protocols, such as GeoJSON or CSV, facilitates this alignment, ensuring that data from various sensors is processed and visualized effectively within GIS systems. This alignment is essential for accurate spatial analysis and decision-making.
Sensor coverage poses another challenge. Fixed-location stations often miss local pollution variations. Deploying a network of IoT sensors enhances spatial resolution, providing comprehensive coverage of urban areas. This expansion improves the granularity of data collection, allowing urban planners to capture detailed pollution patterns.
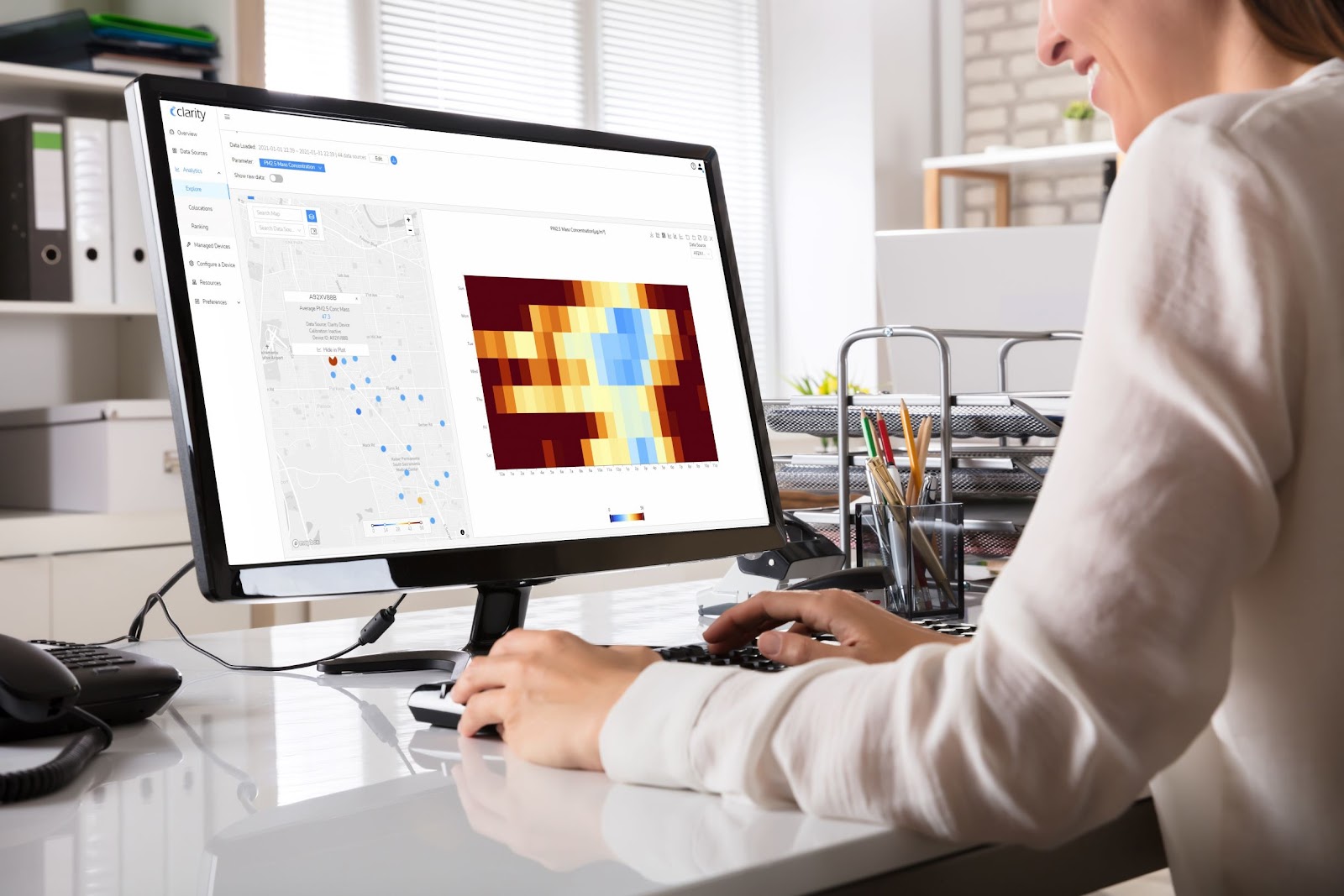
Clarity's Air Quality Management 2.0 approach in integrating diverse datasets supports urban planners in overcoming these challenges. Our solutions ensure that air quality data is accurate, comprehensive, and actionable, aiding informed urban planning and sustainable development.
Leveraging advanced technologies for better integration
Advanced technologies like AI and cloud computing are transforming how air quality data integrates with GIS platforms. These technologies enhance data centralization, allowing urban planners to access comprehensive datasets seamlessly. AI algorithms process vast amounts of air quality data, identifying patterns and trends that may go unnoticed through manual analysis. Cloud computing offers scalable storage solutions, enabling efficient data management and retrieval.
AI's role in data analysis is pivotal. Machine learning models analyze historical air quality data, predicting future trends and providing actionable insights for urban planning. This predictive capability supports proactive decision-making, allowing cities to address potential air quality issues before they escalate. AI enhances the precision of data interpretation, equipping planners with the tools needed for effective urban management—benefits further explored in our detailed data analysis services.
Cloud computing enhances data accessibility. By centralizing data storage in the cloud, urban planners access real-time air quality information from anywhere, facilitating swift decision-making. Cloud platforms provide the computational power necessary to handle large datasets, ensuring that data remains up-to-date and readily available for analysis.
Our expertise in leveraging these technologies empowers urban planners to integrate air quality data with GIS effectively. This integration fosters informed, data-driven planning for sustainable urban development.
Benefits of GIS-enabled air quality monitoring
GIS-enabled systems transform air quality monitoring with precision and depth. Urban planners access insights that drive smarter city development and policy making. These systems integrate spatial data with real-time air quality information, supporting comprehensive urban management strategies.
GIS platforms enhance urban planning by providing a detailed spatial understanding of pollution dynamics. This enables planners to design urban areas that prioritize air quality improvements and sustainability. Regulatory compliance becomes more manageable with GIS, offering tools to track and meet environmental standards. Authorities gain the ability to monitor adherence to air quality regulations effectively.
Citizen engagement is heightened through visual data representations. GIS facilitates transparent communication of air quality information, empowering communities to participate in environmental decision-making processes. Residents access clear, visual insights into local air quality, fostering informed discussions and actions.
For additional context on how advanced air quality monitoring supports sustainable urban policies, review insights on maximizing the impact of environmental policies through enhanced air quality monitoring. Clarity's expertise in integrating GIS with air quality data supports urban planners in achieving these benefits, leading to healthier, more sustainable urban environments.
Key takeaways for effective integration
Integrating air quality data with GIS systems enhances urban planning and environmental management. Real-time data collection, spatial analysis, and community involvement lead to better decisions. Collaborating with researchers, using IoT technology, and maintaining data quality through calibration provides accurate insights. These strategies support sustainable urban development and public health initiatives.
Good practices make air quality monitoring complete and efficient. Partnerships with academic institutions and open data sources improve data quality. When communities help interpret data, policies reflect local needs better. Cost-effective technologies like low-cost sensors help cities monitor air quality comprehensively.
These practices give urban planners insights for better development decisions. See how our air quality solutions can help you. Get a quote today.